Dr. David Greenberg
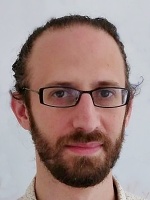
Dr. David Greenberg
Modellbasiertes Maschinelles Lernen
AbteilungsleiterTel: +49 (0) 4152 87-2133
Research
I am interested in what machine learning (ML) can do for the geosciences. Beyond simple problems of predicting, completing or classifying data, I believe ML has significant untapped potential for improving how numerical simulations are developed and used. In particular, parameter tuning, parametrization development, uncertainty quantification and data assimilation are all tasks where a hybrid approach combining the data-efficiency and flexibility of ML with the inductive biases of physics, chemistry and biology can be immensely helpful. Conversely, I think the geosciences also have a great deal to teach ML, and I’m excited by the conceptual and technical challenges raised by the sheer scale of geoscientific datasets and simulations.
- Rubbens, P., Brodie, S., Cordier, T., Destro Barcellos, D., Devos, P., Fernandes-Salvador, J.A., Fincham, J.I., Gomes, A., Handegard, N.O., Howell, K., Jamet, C., Kartveit, K.H., Moustahfid, H., Parcerisas, C., Politikos, D., Sauzède, R., Sokolova, M., Uusitalo, L., Van den Bulcke, L., van Helmond, A.T.M., Watson, J.T., Welch, H., Beltran-Perez, O., Chaffron, S., Greenberg, D.S., Kühn, B., Kiko, R., Lo, M., Lopes, R.M., Möller, K.O., Michaels, W., Pala, A., Romagnan, J.-B., Schuchert, P., Seydi, V., Villasante, S., Malde, K., & Irisson, J.-O. (2023): ICES Journal of Marine Science, 2023, fsad100, doi:10.1093/icesjms/fsad100
- Schanz, T., Möller, K. O., Rühl, S., & Greenberg, D. S. (2023): Robust detection of marine life with label-free image feature learning and probability calibration. Machine Learning: Science and Technology, 4(3), 035007, doi:10.1088/2632-2153/ace417
- Paasche, H., Gross, M., Lüttgau, J., Greenberg, D.S., & Weigel, T. (2022): To the brave scientists: Aren't we strong enough to stand (and profit from) uncertainty in Earth system measurement and modelling? Geoscience Data Journal, 9, 393– 399, doi:10.1002/gdj3.132
- Ramesh, P., Lueckmann, J.-M., Boelts, J., Tejero-Cantero, A., Greenberg, D.S., Gonçalves, P.J., & Macke, J.H. (2022): GATSBI: Generative Adversarial Training for Simulation-Based Inference. arXivLabs, Machine Learning, doi:10.48550/arXiv.2203.06481
- Lueckmann, Jan-Matthis, Boelts, Jan, Greenberg, David, Goncalves, Pedro, Macke, Jakob (2021): Benchmarking Simulation-Based Inference. Proceedings of The 24th International Conference on Artificial Intelligence and Statistics, PMLR 130:343-351, 2021
- Nonnenmacher, M., & Greenberg, D.S. (2021): Deep emulators for differentiation, forecasting, and parametrization in Earth science simulators. Journal of Advances in Modeling Earth Systems, 13, e2021MS002554, doi:10.1029/2021MS002554
- Nonnenmacher, Marcel, and David S. Greenberg. "Learning Implicit PDE Integration with Linear Implicit Layers."The Symbiosis of Deep Learning and Differential Equations, Neurips 2021
- Paasche, Hendrik, Gross, Matthias, Lüttgau, Jakob, Greenberg, David, Weigel, Tobias (2021). To the brave scientists: Aren't we strong enough to stand (and profit from) uncertainty in Earth system measurement and modelling?. Geoscience Data Journal. 10.1002/gdj3.132
- Gonçalves, P. J., Lueckmann, J. M., Deistler, M., Nonnenmacher, M., Öcal, K., Bassetto, G., Chintaluri, C., Podlaski, W. F., Haddad, S. A., Vogels, T. P., Greenberg, D. S., & Macke, J. H. (2020). Training deep neural density estimators to identify mechanistic models of neural dynamics. eLife, 9, e56261. doi:10.7554/eLife.56261
- Tejero-Cantero, A.; Boelts, J.; Deistler, M.; Lueckmann, J.; Durkan, C.; Goncalves, P.; Greenberg, D.; Macke, J.: sbi: A toolkit for simulation-based inference. In: The Journal of Open Source Software. Vol. 5 (2020) 52, 2505, DOI: /10.21105/joss.0250